Self-learning Features In Smart Devices
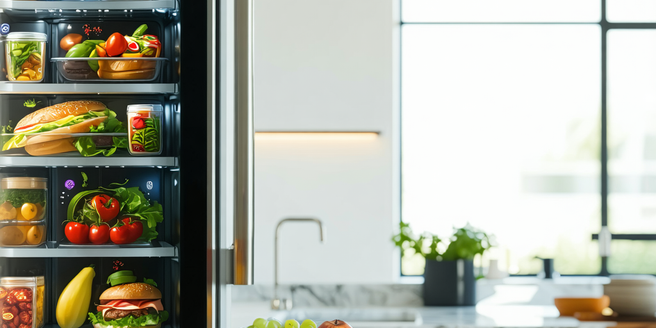
Introduction to Self-learning in Technology
Self-learning in technology marks a pivotal shift, where devices autonomously improve their functionality through user interactions. This ability enables systems to adapt and respond intelligently to varying conditions without explicit programming. In essence, self-learning technologies harness data and computational power to evolve from static machines into dynamic tools that predict and cater to human needs. The advent of these technologies has been transformative, reshaping industries and introducing new possibilities for personalization and efficiency. By integrating algorithms designed to recognize patterns and learn from them, these smart systems make real-time decisions that enhance user experience. Today, sectors such as healthcare, automotive, and home automation are at the forefront, leveraging these advancements for innovation that promises to revolutionize modern living and redefine human-technology interaction.
The Role of Artificial Intelligence in Self-learning
Artificial Intelligence (AI) serves as the backbone of self-learning systems. AI algorithms, especially those rooted in machine learning, empower devices to analyze vast amounts of data swiftly and accurately. This capability allows them to evolve based on previous interactions and new information. As AI technology advances, it integrates increasingly sophisticated methods to enable machines to function and think similarly to humans. AI’s contribution lies in the creation of intelligent agents, which can autonomously make decisions, process natural language, and understand visual inputs. Consequently, AI transforms ordinary devices into smart, self-sufficient entities, capable of predictive analysis and automation. Thus, AI is not just instrumental in advancing self-learning but is also a catalyst for the next wave of technological innovation, defining the future of smart interactions.
How Smart Devices Adapt to User Preferences
Smart devices increasingly redefine user interaction by learning and adapting to preferences and habits. Through continuous data collection and pattern analysis, these devices tailor their responses to individual user needs. For instance, smart thermostats learn preferred temperatures at particular times, while mobile apps suggest content based on previous behavior. This personalization arises from a combination of sensors and machine learning algorithms that interpret user data to deliver customized experiences. Such adaptation enhances user satisfaction by creating a seamless interface that evolves according to personal preferences. Over time, the more data these devices gather, the more precise and intuitive their responses become. As a result, users benefit from convenience and comfort previously unattainable without human intervention, reflecting a prominent shift in the user-device dynamic.
Impact of Self-learning on User Privacy and Security
While self-learning features offer enhanced user experiences, they also raise significant privacy and security concerns. As devices gather extensive personal data to optimize functionality, the risk of data breaches and unauthorized access escalates. Ensuring user privacy necessitates sophisticated encryption and robust security protocols. Additionally, transparency in data usage policies is critical for building trust with consumers. Users must be informed about how their data is collected, stored, and utilized. Cybersecurity measures must evolve alongside technology to keep pace with potential vulnerabilities. Ethical considerations also arise regarding the extent to which devices should analyze personal information. Evaluating and mitigating these risks are essential for the responsible deployment of self-learning technologies, ensuring that innovation does not come at the expense of user security and privacy.
The Future of Self-learning in Smart Devices
The future of self-learning in smart devices is promising, with continuous advancements steering towards a fully automated and intelligent environment. Emerging technologies like edge computing and 5G will significantly enhance processing speeds and device capabilities. This evolution will allow devices to offer real-time insights and actions without latency, increasing their utility and precision. In the coming years, we can anticipate more intuitive interfaces, where devices not only respond to but also anticipate user needs proactively. Integration with the Internet of Things (IoT) will offer even more opportunities for interconnected solutions, facilitating cohesive smart ecosystems. As these technologies develop, they will likely permeate every aspect of daily life, from healthcare to urban planning, promising a future defined by efficiency and an unprecedented level of personalization in technological interactions.